GEP fellow publishes RNA splicing study
Jamie Ellingford and his co-authors explore the potential of computational tools to help us learn more about the messages our genes create
Predictive technologies can be vital to help diagnose – or refine existing diagnoses – of patients with rare genetic conditions.
Below, Genomics Education Programme fellow Jamie Ellingford talks to us about his new research focusing on genomic variants that disrupt RNA splicing and which computational tools can most accurately identify them.
What is splicing?
The human genome is made up of about 20,000 genes that contain the messages to create proteins and enable cells and tissues to grow and function properly. Most human genes have parts called introns that need to be cut out so that messages can be communicated appropriately. When this process (termed ‘splicing’) is disrupted, it can lead to errors in the messages that the genes create, and this can lead to disease in some cases.
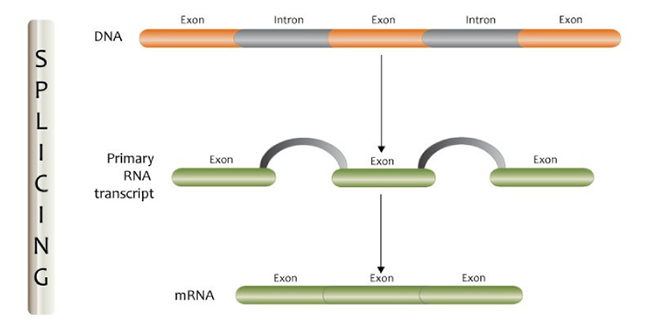
The process of splicing. Image from GEP Flickr channel.
One of the major challenges associated with analysing the complete human genome is our ability to identify individual differences (‘genomic variants’) that lead to these types of disruption.
In this study, we assessed several different computational approaches to identify variants that are disruptive to the process of splicing.
Comparing predictive tools
We first identified a set of genomic variants that we were confident either disrupted normal splicing or not, and then obtained this information for 249 genomic variants identified in individuals with a range of genetic disorders.
We then compared different computational prediction tools to assess how accurately they could identify the genomic variants that we had found to have an impact.
Our research showed that one of the computational tools, ‘SpliceAI’, worked best as a standalone computational approach, but that combining the predictions from all tools offered some advantages in accuracy.
Use in diagnostic genetic testing
Next, we wanted to see the impact that incorporating these computational strategies would have on diagnostic genetic testing.
We did this for 2783 individuals with rare genetic conditions and observed that this could lead to a new or refined diagnosis in at least 3% of individuals.
This uplift was a result of the identification of genomic variants that had not been prioritised from standard diagnostic genetic testing approaches.
Complex and unexpected impacts
During our analysis, we looked in detail at the specific impact genomic variants had on the messages that were created from genes.
The intermediate molecule between DNA and protein (messenger RNA, or mRNA) can be accessed directly from blood or cells to assess how it has been altered in the presence of genomic variants, or we can create artificially models in controlled experiments.
In some cases, we observed the mRNA was impacted in a complex and surprising manner which was not predicted from the computational tools.
Conclusions
Some computational tools are highly accurate for the prioritisation of genomic variants that impact splicing, but to accurately work out the exact impact of these variants, we need to have approaches to undertake detailed investigations of the messages that genes create.
The integration of these approaches into diagnostic genetic services can lead to an increase in the number of genetic diagnoses identified, and, in the future, may enable direct and targeted treatment of these disruptions.
The study is available to read as an open access article in Nature
Read more about Jamie and his project, along with information about our other fellows, on our website
–